Probability & Statistics
Research in Probability and Statistics at McMaster is highly interdisciplinary, and reaches beyond the department into the Faculties of Science, Medical, Engineering and Business. Research interests include: Theoretical Probability (large deviations, stochastic differential equations, martingales) Applied Probability (stochastic genetics, queueing systems) and Mathematical Statistics (inferential methods, model-validity, outliers, multivariate analysis) and Applied Statistics (environmental hazards, animal abundance, genetics, climatology, pharmaceutics, and mortality).
Information Box Group
N. Balakrishnan
Distinguished University Professor
Research Area: probability-statistics
Research Profile: Order statistics, distribution theory.
My research interests are in order statistics, outliers, distribution theory, statistical inference, records, multivariate analysis, and industrial statistics. Order statistics arise naturally in many problems such as life-testing and reliability experiments, outlier detection, robust inference, etc. My work in this area covers both theoretical (like bounds, approximations, recurrence relations, characterizations, etc.) as well as applied (like linear inference, acceptance sampling plans, tolerance limits, prediction, etc.) aspects. Another major area of interest to me is statistical distribution theory. Statistical distributions play a fundamental role in many applied problems and form the basis for model building and parametric statistical inference. My contributions to this area have been through proposing new distributions, developing inference techniques for several distributions, and deriving characterizations of distributions. My most recent work concerns order statistics under non-standard conditions. Work in this direction is carried out by using permanents (of some special matrices) and their properties.
Ben Bolker
Professor, Director of School of Computational Science and Engineering and Associate Chair (Graduate)
Research Area: mathematical-biology
Research Profile: mathematical-biology.
I work on a wide variety of problems in theoretical ecology and evolution. Mathematical interests: the use of “moment equations” (equations for the mean, variance, and second-order spatial statistics such as spatial correlation) to understand the qualitative dynamics of biological communities (e.g. hosts and parasites, competing plant species)interacting in continuous spatial arenas. Biological interests: host/parasite interactions, broadly defined to include any “intimate association” among natural enemies and their victims: viruses, bacteria, and classical macroparasites. I work on the general dynamics of infectious disease epidemics, and particularly on the evolution of virulence. Statistical interests: fitting of parameters for ecological and evolutionary models, especially in a maximum likelihood settings. My recent work has focused on improvement and dissemination of tools for fitting generalized linear mixed models.
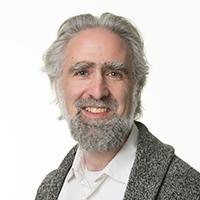
Ben Bolker
Professor, Director of School of Computational Science and Engineering and Associate Chair (Graduate)
Angelo Canty
Associate Professor
Research Area: probability-statistics
Research Profile: Computational statistics
My research focuses on the applied uses of computationally intensive methods in statistics. One area in which computaional power is particularly useful is in the use of Monte Carlo approximations which can replace intractable calculations or asymptotic argeuments. The use of these methods, however, require reliable software and good diagnostics for when there may be problems with the underling assumptions. In my doctoral work I examined diagnostics for Markov chain Monte Carlo methods which are particularly important in Bayesian statistics. Since then I have worked on the bootstrap and other resampling methods. A suite of functions that I have developed to implement many of these methods is used worldwide. These functions allow for the application of resampling methods in many fields. Much remains to be done, however. I am currently working on applied graphical diagnostics for bootstrap failure. I am also examining new areas of application for these methods and improvements on existing methods. My latest area of research is in Monte Carlo implementations of the EM algorithm for likelihood inference when more standard methods cannot be used.
Aaron Childs
Associate Professor and TA Coordinator
Research Area: probability-statistics
Research Profile: Statistical Inference, order statistics, outliers
I am interested in the diverse applications of order statistics to statistical inference and outlier accomodation. Some of my research involves order statistics from independent and non-identically distributed (I.NI.D) random variables. I.NI.D. random variables are a generalization of the multiple-outlier slippage model, and therefore have applications to outlier accomodation. Order statistics also have applications in classical inference problems; for example I have developed some hypothesis tests based on order statistics and inverse sampling which provide a more efficient alternative to the standard chi-square test for homogeneity. I have also developed a set of Maple programs that allow one to compute series approximations for moments of order statistics. Another area of interest to me is waiting time problems. My contribution to this area has been to develop new methods, some of which are based on uniform random variables and generating functions, which enable one to solve a wide variety of very complex waiting time problems. I have also been involved in some consulting projects, one of which requires testing for independence between virus and respiratory disease data using time series analysis.
Katherine Davies
Assistant Professor
Research Area: Probabiility and Statistics
Shui Feng
Professor and Associate Chair (Statistics)
Research Area: probability-statistics
Research Profile: Stochastic processes, interacting particle systems
My research interests are in nonlinear stochastic model, large deviation, hydrodynamic limit and stochastic models in genetics. Some concrete problems are the long-time behaviour, the phase structure and metastability of the corresponding system. The method used comes from probability theory, stochastic processes and stochastic analysis.
Noah Forman
Associate Professor
I specialize in probability theory, specifically in combinatorial stochastic processes. This means studying random objects and processes on combinatorial spaces, such as random graphs, as well as studying continuum scaling limits or analogues of these objects. Much of my research concerns exchangeability and continuum random trees.
Pratheepa Jeganathan
Assistant Professor
Reserach Area: Statistics and data mining
Research Profile: Statistics and data mining
Dr. Jeganathan’s research focuses on developing statistical and computational methods to analyze multi-domain data, especially addressing statistical challenges in microbiome multi-omic and spatial multi-omic data analysis. Current research includes multi-table integration, preprocessing and transformation of high-throughput sequencing data, visualization, hierarchical modeling, Bayesian statistics, statistical inference, block bootstrap method, data mining, and approximation theory in statistics.
Sharon King-Yu
Assistant Professor
Research Area: Computational Statistics
Paul McNicholas
Professor, Canada Research Chair
Research Area: Computational Statistics
Research Profile: Computational Statistics
Dr. McNicholas’ research focuses on computational statistics, and he is at the cutting edge of international research on mixture model-based clustering and classification. Current research includes work on big data featuring outlying or spurious points, with a focus on classification, clustering, dimension reduction and discriminant analysis. Another important aspect of Dr. McNicholas? current research is work on non-Gaussian mixture models, which present a useful alternative to the Gaussian mixture model. Work on clustering categorical data and data of mixed type is ongoing. Applications of Dr. McNicholas? research are readily found in several fields, including bioinformatics, sensometrics, and psychometrics.
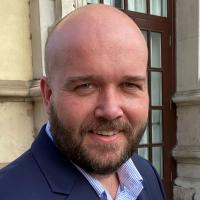
Paul McNicholas
Professor, Canada Research Chair
N. Balakrishnan
Distinguished University Professor
Research Area: probability-statistics
Research Profile: Order statistics, distribution theory.
My research interests are in order statistics, outliers, distribution theory, statistical inference, records, multivariate analysis, and industrial statistics. Order statistics arise naturally in many problems such as life-testing and reliability experiments, outlier detection, robust inference, etc. My work in this area covers both theoretical (like bounds, approximations, recurrence relations, characterizations, etc.) as well as applied (like linear inference, acceptance sampling plans, tolerance limits, prediction, etc.) aspects. Another major area of interest to me is statistical distribution theory. Statistical distributions play a fundamental role in many applied problems and form the basis for model building and parametric statistical inference. My contributions to this area have been through proposing new distributions, developing inference techniques for several distributions, and deriving characterizations of distributions. My most recent work concerns order statistics under non-standard conditions. Work in this direction is carried out by using permanents (of some special matrices) and their properties.
N. Balakrishnan
Distinguished University Professor
Research Area: probability-statistics
Research Profile: Order statistics, distribution theory.
My research interests are in order statistics, outliers, distribution theory, statistical inference, records, multivariate analysis, and industrial statistics. Order statistics arise naturally in many problems such as life-testing and reliability experiments, outlier detection, robust inference, etc. My work in this area covers both theoretical (like bounds, approximations, recurrence relations, characterizations, etc.) as well as applied (like linear inference, acceptance sampling plans, tolerance limits, prediction, etc.) aspects. Another major area of interest to me is statistical distribution theory. Statistical distributions play a fundamental role in many applied problems and form the basis for model building and parametric statistical inference. My contributions to this area have been through proposing new distributions, developing inference techniques for several distributions, and deriving characterizations of distributions. My most recent work concerns order statistics under non-standard conditions. Work in this direction is carried out by using permanents (of some special matrices) and their properties.
Ben Bolker
Professor, Director of School of Computational Science and Engineering and Associate Chair (Graduate)
Research Area: mathematical-biology
Research Profile: mathematical-biology.
I work on a wide variety of problems in theoretical ecology and evolution. Mathematical interests: the use of “moment equations” (equations for the mean, variance, and second-order spatial statistics such as spatial correlation) to understand the qualitative dynamics of biological communities (e.g. hosts and parasites, competing plant species)interacting in continuous spatial arenas. Biological interests: host/parasite interactions, broadly defined to include any “intimate association” among natural enemies and their victims: viruses, bacteria, and classical macroparasites. I work on the general dynamics of infectious disease epidemics, and particularly on the evolution of virulence. Statistical interests: fitting of parameters for ecological and evolutionary models, especially in a maximum likelihood settings. My recent work has focused on improvement and dissemination of tools for fitting generalized linear mixed models.
Ben Bolker
Professor, Director of School of Computational Science and Engineering and Associate Chair (Graduate)
Research Area: mathematical-biology
Research Profile: mathematical-biology.
I work on a wide variety of problems in theoretical ecology and evolution. Mathematical interests: the use of “moment equations” (equations for the mean, variance, and second-order spatial statistics such as spatial correlation) to understand the qualitative dynamics of biological communities (e.g. hosts and parasites, competing plant species)interacting in continuous spatial arenas. Biological interests: host/parasite interactions, broadly defined to include any “intimate association” among natural enemies and their victims: viruses, bacteria, and classical macroparasites. I work on the general dynamics of infectious disease epidemics, and particularly on the evolution of virulence. Statistical interests: fitting of parameters for ecological and evolutionary models, especially in a maximum likelihood settings. My recent work has focused on improvement and dissemination of tools for fitting generalized linear mixed models.
Angelo Canty
Associate Professor
Research Area: probability-statistics
Research Profile: Computational statistics
My research focuses on the applied uses of computationally intensive methods in statistics. One area in which computaional power is particularly useful is in the use of Monte Carlo approximations which can replace intractable calculations or asymptotic argeuments. The use of these methods, however, require reliable software and good diagnostics for when there may be problems with the underling assumptions. In my doctoral work I examined diagnostics for Markov chain Monte Carlo methods which are particularly important in Bayesian statistics. Since then I have worked on the bootstrap and other resampling methods. A suite of functions that I have developed to implement many of these methods is used worldwide. These functions allow for the application of resampling methods in many fields. Much remains to be done, however. I am currently working on applied graphical diagnostics for bootstrap failure. I am also examining new areas of application for these methods and improvements on existing methods. My latest area of research is in Monte Carlo implementations of the EM algorithm for likelihood inference when more standard methods cannot be used.
Angelo Canty
Associate Professor
Research Area: probability-statistics
Research Profile: Computational statistics
My research focuses on the applied uses of computationally intensive methods in statistics. One area in which computaional power is particularly useful is in the use of Monte Carlo approximations which can replace intractable calculations or asymptotic argeuments. The use of these methods, however, require reliable software and good diagnostics for when there may be problems with the underling assumptions. In my doctoral work I examined diagnostics for Markov chain Monte Carlo methods which are particularly important in Bayesian statistics. Since then I have worked on the bootstrap and other resampling methods. A suite of functions that I have developed to implement many of these methods is used worldwide. These functions allow for the application of resampling methods in many fields. Much remains to be done, however. I am currently working on applied graphical diagnostics for bootstrap failure. I am also examining new areas of application for these methods and improvements on existing methods. My latest area of research is in Monte Carlo implementations of the EM algorithm for likelihood inference when more standard methods cannot be used.
Aaron Childs
Associate Professor and TA Coordinator
Research Area: probability-statistics
Research Profile: Statistical Inference, order statistics, outliers
I am interested in the diverse applications of order statistics to statistical inference and outlier accomodation. Some of my research involves order statistics from independent and non-identically distributed (I.NI.D) random variables. I.NI.D. random variables are a generalization of the multiple-outlier slippage model, and therefore have applications to outlier accomodation. Order statistics also have applications in classical inference problems; for example I have developed some hypothesis tests based on order statistics and inverse sampling which provide a more efficient alternative to the standard chi-square test for homogeneity. I have also developed a set of Maple programs that allow one to compute series approximations for moments of order statistics. Another area of interest to me is waiting time problems. My contribution to this area has been to develop new methods, some of which are based on uniform random variables and generating functions, which enable one to solve a wide variety of very complex waiting time problems. I have also been involved in some consulting projects, one of which requires testing for independence between virus and respiratory disease data using time series analysis.
Aaron Childs
Associate Professor and TA Coordinator
Research Area: probability-statistics
Research Profile: Statistical Inference, order statistics, outliers
I am interested in the diverse applications of order statistics to statistical inference and outlier accomodation. Some of my research involves order statistics from independent and non-identically distributed (I.NI.D) random variables. I.NI.D. random variables are a generalization of the multiple-outlier slippage model, and therefore have applications to outlier accomodation. Order statistics also have applications in classical inference problems; for example I have developed some hypothesis tests based on order statistics and inverse sampling which provide a more efficient alternative to the standard chi-square test for homogeneity. I have also developed a set of Maple programs that allow one to compute series approximations for moments of order statistics. Another area of interest to me is waiting time problems. My contribution to this area has been to develop new methods, some of which are based on uniform random variables and generating functions, which enable one to solve a wide variety of very complex waiting time problems. I have also been involved in some consulting projects, one of which requires testing for independence between virus and respiratory disease data using time series analysis.
Katherine Davies
Assistant Professor
Research Area: Probabiility and Statistics
Katherine Davies
Assistant Professor
Research Area: Probabiility and Statistics
Shui Feng
Professor and Associate Chair (Statistics)
Research Area: probability-statistics
Research Profile: Stochastic processes, interacting particle systems
My research interests are in nonlinear stochastic model, large deviation, hydrodynamic limit and stochastic models in genetics. Some concrete problems are the long-time behaviour, the phase structure and metastability of the corresponding system. The method used comes from probability theory, stochastic processes and stochastic analysis.
Shui Feng
Professor and Associate Chair (Statistics)
Research Area: probability-statistics
Research Profile: Stochastic processes, interacting particle systems
My research interests are in nonlinear stochastic model, large deviation, hydrodynamic limit and stochastic models in genetics. Some concrete problems are the long-time behaviour, the phase structure and metastability of the corresponding system. The method used comes from probability theory, stochastic processes and stochastic analysis.
Noah Forman
Associate Professor
I specialize in probability theory, specifically in combinatorial stochastic processes. This means studying random objects and processes on combinatorial spaces, such as random graphs, as well as studying continuum scaling limits or analogues of these objects. Much of my research concerns exchangeability and continuum random trees.
Noah Forman
Associate Professor
I specialize in probability theory, specifically in combinatorial stochastic processes. This means studying random objects and processes on combinatorial spaces, such as random graphs, as well as studying continuum scaling limits or analogues of these objects. Much of my research concerns exchangeability and continuum random trees.
Pratheepa Jeganathan
Assistant Professor
Reserach Area: Statistics and data mining
Research Profile: Statistics and data mining
Dr. Jeganathan’s research focuses on developing statistical and computational methods to analyze multi-domain data, especially addressing statistical challenges in microbiome multi-omic and spatial multi-omic data analysis. Current research includes multi-table integration, preprocessing and transformation of high-throughput sequencing data, visualization, hierarchical modeling, Bayesian statistics, statistical inference, block bootstrap method, data mining, and approximation theory in statistics.
Pratheepa Jeganathan
Assistant Professor
Reserach Area: Statistics and data mining
Research Profile: Statistics and data mining
Dr. Jeganathan’s research focuses on developing statistical and computational methods to analyze multi-domain data, especially addressing statistical challenges in microbiome multi-omic and spatial multi-omic data analysis. Current research includes multi-table integration, preprocessing and transformation of high-throughput sequencing data, visualization, hierarchical modeling, Bayesian statistics, statistical inference, block bootstrap method, data mining, and approximation theory in statistics.
Sharon King-Yu
Assistant Professor
Research Area: Computational Statistics
Sharon King-Yu
Assistant Professor
Research Area: Computational Statistics
Paul McNicholas
Professor, Canada Research Chair
Research Area: Computational Statistics
Research Profile: Computational Statistics
Dr. McNicholas’ research focuses on computational statistics, and he is at the cutting edge of international research on mixture model-based clustering and classification. Current research includes work on big data featuring outlying or spurious points, with a focus on classification, clustering, dimension reduction and discriminant analysis. Another important aspect of Dr. McNicholas? current research is work on non-Gaussian mixture models, which present a useful alternative to the Gaussian mixture model. Work on clustering categorical data and data of mixed type is ongoing. Applications of Dr. McNicholas? research are readily found in several fields, including bioinformatics, sensometrics, and psychometrics.
Paul McNicholas
Professor, Canada Research Chair
Research Area: Computational Statistics
Research Profile: Computational Statistics
Dr. McNicholas’ research focuses on computational statistics, and he is at the cutting edge of international research on mixture model-based clustering and classification. Current research includes work on big data featuring outlying or spurious points, with a focus on classification, clustering, dimension reduction and discriminant analysis. Another important aspect of Dr. McNicholas? current research is work on non-Gaussian mixture models, which present a useful alternative to the Gaussian mixture model. Work on clustering categorical data and data of mixed type is ongoing. Applications of Dr. McNicholas? research are readily found in several fields, including bioinformatics, sensometrics, and psychometrics.